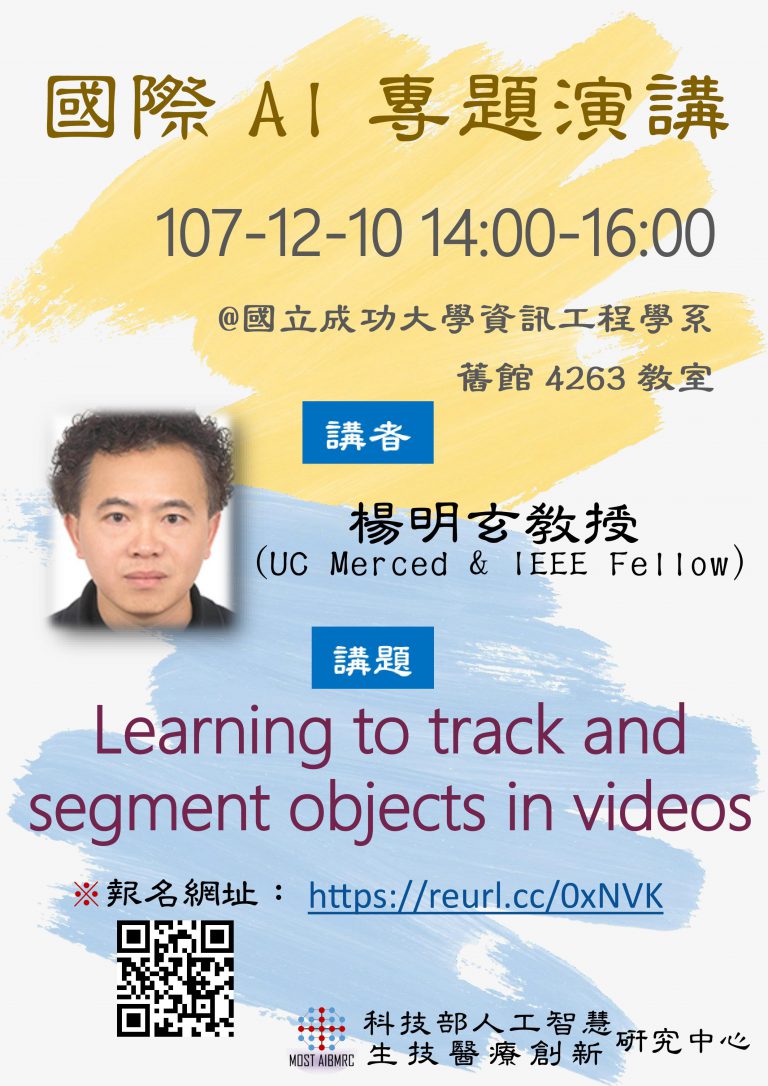
日期:107年12月10日
時間:14:00-16:00
地點:國立成功大學資訊工程學系舊館4263教室
演講者:楊明玄(UC Merced)
講題:Learning to track and segment objects in videos
報名網址:https://reurl.cc/0xNVK
講者簡歷
Ming-Hsuan Yang is a research scientist at Google Cloud and a professor in Electrical Engineering and Computer Science at University of California, Merced. He is elevated to IEEE Fellow in January 2019.
講題摘要
The tracking-by-detection framework typically consists of two stages, i.e., drawing samples around the target object in the first stage and classifying each sample as the target object or as background in the second stage. The performance of existing trackers using deep classification networks is limited by two aspects. First, the positive samples in each frame are highly spatially overlapped, and they fail to capture rich appearance variations. Second, there exists extreme class imbalance between positive and negative samples. This VITAL algorithm aims to address these two problems via adversarial learning. To augment positive samples, we use a generative network to randomly generate masks, which are applied to adaptively dropout input features to capture a variety of appearance changes. With the use of adversarial learning, our network identifies the mask that maintains the most robust features of the target objects over a long temporal span. In addition, to handle the issue of class imbalance, we propose a high-order cost sensitive loss to decrease the effect of easy negative samples to facilitate training the classification network. Extensive experiments on benchmark datasets demonstrate that the proposed tracker performs favorably against the state-of-the-art approaches.
Online video object segmentation is a challenging task as it entails to process the image sequence timely and accurately. To segment a target object through the video, numerous CNN-based methods have been developed by heavily fine-tuning on the object mask in the first frame, which is time-consuming for online applications. In the second part, we propose a fast and accurate video object segmentation algorithm that can immediately start the segmentation process once receiving the images. We first utilize a part-based tracking method to deal with challenging factors such as large deformation, occlusion, and cluttered background.