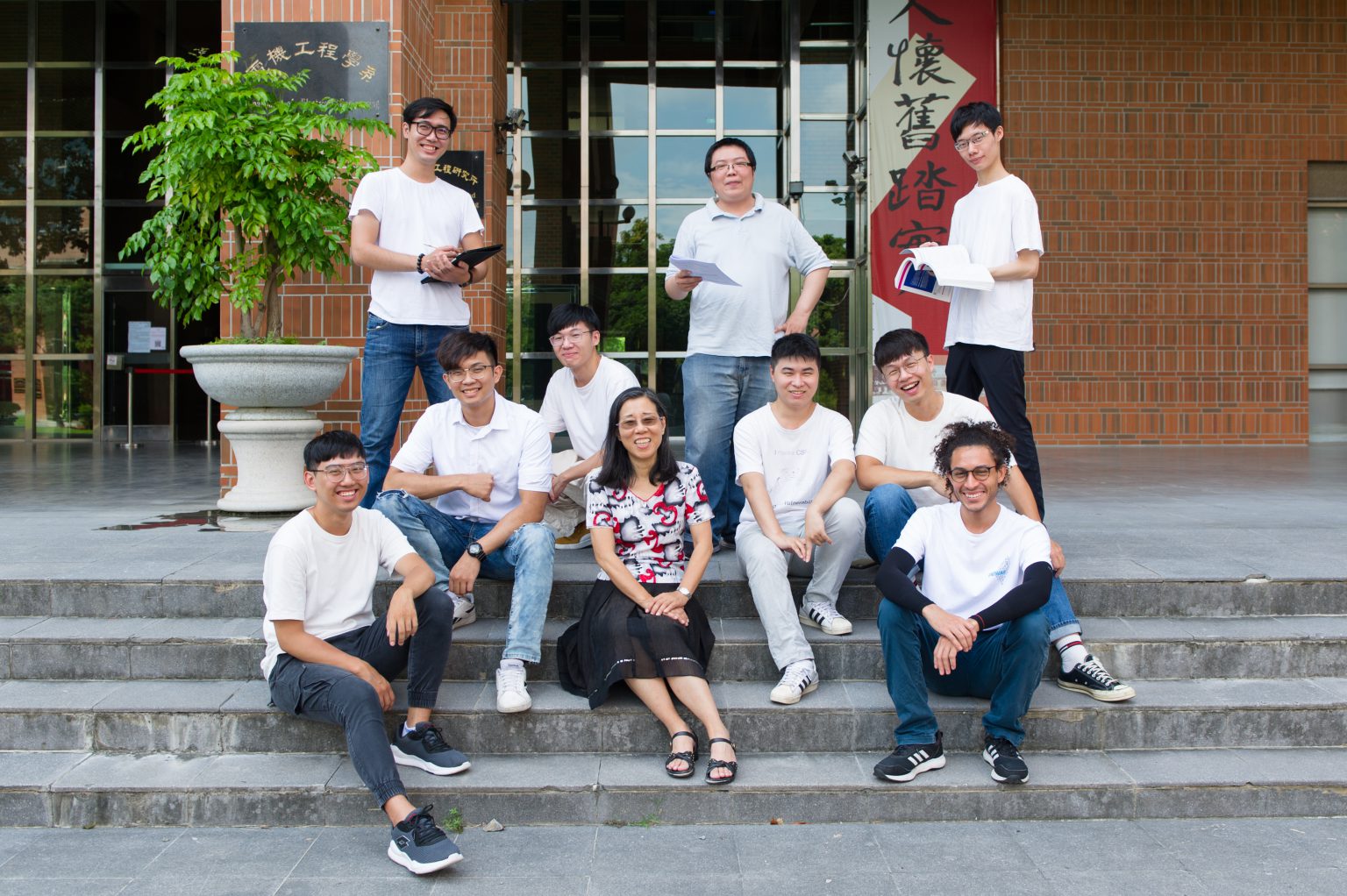
AI Medical Devices: Liver Biopsy Image Analysis
Principal Investigator |
Pau-Choo Chung,distinguished Professor
Department of Electrical Engineering
Co-Principal Investigator |
Kuo-Sheng Cheng,Professor Department of Biomedical Engineering
Chow, Nan-Haw,Professor Department of Pathology, College of Medicine, National Cheng Kung University
Hung-Wen, Tsai,Associate Professor Department of Pathology, College of Medicine, National Cheng Kung University
Tseng-Lung YANG Kaohsiung Veterans General Hospital
Kuo-Sheng Cheng,Professor Department of Biomedical Engineering
Chow, Nan-Haw,Professor Department of Pathology, College of Medicine, National Cheng Kung University
Hung-Wen, Tsai,Associate Professor Department of Pathology, College of Medicine, National Cheng Kung University
Tseng-Lung YANG Kaohsiung Veterans General Hospital
Project Intro
Liver histopathology image analysis is a commonly used approach to confirm the decision in liver diseases diagnosis. Current assistive histopathology image analysis systems have their function limitations in extracting morphology features, effective discrimination of cancer and normal tissues. Furthermore, some disease types of cells have highly varied features and ambiguous boundary. Some region segmentation such as the portal area segmentation, also need to refer to surrounding cells. All of these characteristics make the analysis of liver histopathology image very challenging. In view of these limitations, this project aims to develop new deep learning modules, combined with image analysis, for the analysis of liver histopathology images, the detection of liver cancers, the grading of inflammation degrees. The results will be realized into a practical system for doctors to test and provide feedback, for achieving the precision medicine.
Liver histopathology image analysis is a commonly used approach to confirm the decision in liver diseases diagnosis. Current assistive histopathology image analysis systems have their function limitations in extracting morphology features, effective discrimination of cancer and normal tissues. Furthermore, some disease types of cells have highly varied features and ambiguous boundary. Some region segmentation such as the portal area segmentation, also need to refer to surrounding cells. All of these characteristics make the analysis of liver histopathology image very challenging. In view of these limitations, this project aims to develop new deep learning modules, combined with image analysis, for the analysis of liver histopathology images, the detection of liver cancers, the grading of inflammation degrees. The results will be realized into a practical system for doctors to test and provide feedback, for achieving the precision medicine.
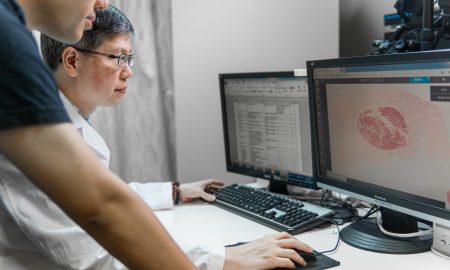
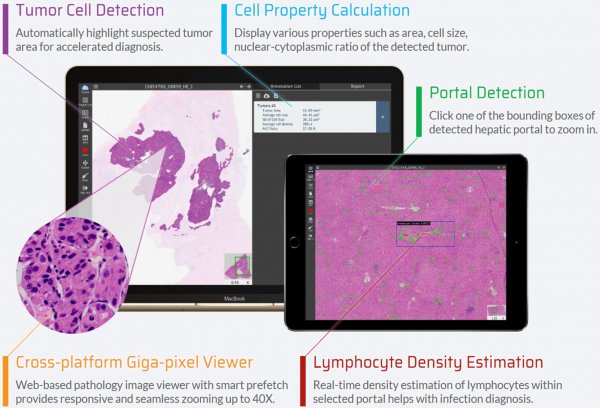
System Capabilities
Our project successfully built a cross-platform pathology cloud, integrating algorithms into the platform for doctors to improve diagnosis progress using these developed AI algorithms.
Our project successfully built a cross-platform pathology cloud, integrating algorithms into the platform for doctors to improve diagnosis progress using these developed AI algorithms.
- Liver Tumor detection and Grading System (Detection Sensitivity = 0.95 Detection IoU = 0.9, Grading Accuracy = 0.8)
- Lymphocyte Detection (F1 Score = 0.92)
- Hepatitis Classification cell segmentation (Avg. IoU = 0.70 , Avg. F1 Score = 0.81)
- portal vein/central vein segmentation
- Fibrosis segmentation
- Tumor Stage Determination
- Cross-platform pathology image browsing, real-time display in different magnifications
- Image calibration
Cooperating Partners
- National Chip Implementation Center
- National Center for High-Performance
- Computing Kaohsiung Veterans General Hospital Origins & Enterprises
- NCKU Hospital
Contact info
Tel :06-2757575 ext.62373
Mail:pcchung@ee.ncku.edu.tw
Tel :06-2757575 ext.62373
Mail:pcchung@ee.ncku.edu.tw
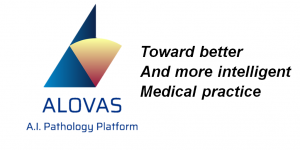