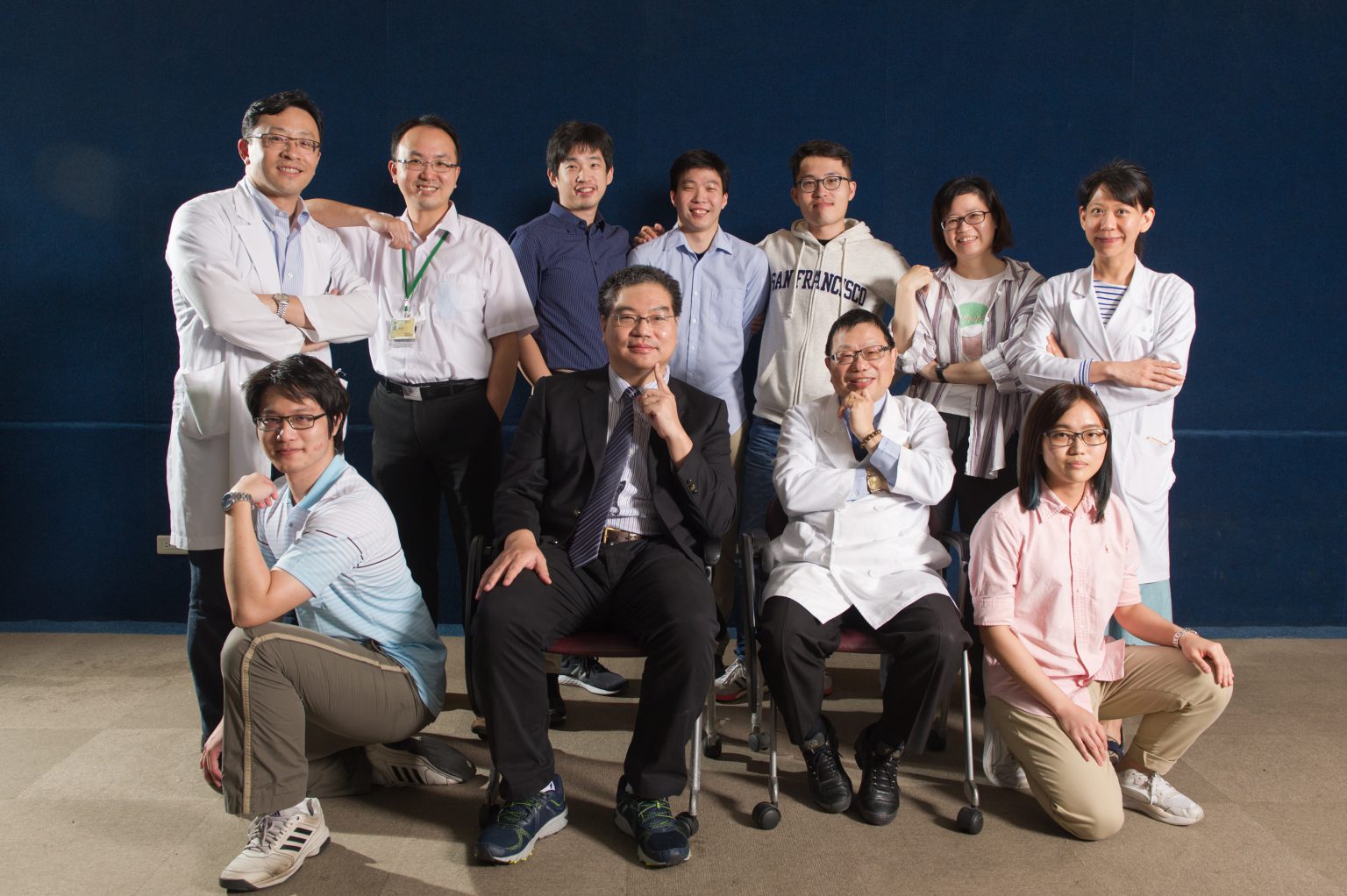
Artificial intelligence driven automatic tumor detection and follow up, and precision medicine model for acoustic neuroma
Principal Investigator |
Yu-Te Wu
Distinguished Professor
Institute of Biophotonics, NYMU
Co-Principal Investigator |
Kun-Pin Wu, Professor Institute of Biomedical Informatics, NYMU
Chia-Feng Lu, Assistant Professor Biomedical Imaging and Radiological Sciences Dept, NYMU (BIRS)
Wan-Yuo Guo、Hsiu-Mei Wu、Chih-Chun Wu, Attending Physician Department of Radiology Taipei Veterans General Hospital
Wen-Yuh Chung、Huai-Zhe Yang、Cheng-chia Lee, Attending Physician Department of Neurosurgery, Neurologial Institute
Li-Bo Hung, Attending Physician Cheng Hsin General Hospital
Po-Shan Wang, Attending Physician Taipei Municipal Gan-Dau Hospital
Michelle Liou, Researcher Institute of Statistical Science, Academia Sinica
Co-Principal Investigator |
Kun-Pin Wu, Professor Institute of Biomedical Informatics, NYMU
Chia-Feng Lu, Assistant Professor Biomedical Imaging and Radiological Sciences Dept, NYMU (BIRS)
Wan-Yuo Guo、Hsiu-Mei Wu、Chih-Chun Wu, Attending Physician Department of Radiology Taipei Veterans General Hospital
Wen-Yuh Chung、Huai-Zhe Yang、Cheng-chia Lee, Attending Physician Department of Neurosurgery, Neurologial Institute
Li-Bo Hung, Attending Physician Cheng Hsin General Hospital
Po-Shan Wang, Attending Physician Taipei Municipal Gan-Dau Hospital
Michelle Liou, Researcher Institute of Statistical Science, Academia Sinica
Project Intro
Vestibular schwannoma (VS) is a benign tumor with slow growth rate and clear boundary. Gamma knife (GK) surgery is a promising treatment for patients with VS. Taipei Veterans General Hospital (TVGH) is an internationally renowned treatment center for its reputation of GK. Thousands of GK cases have been collected in TVGH, including 500 VS cases of complete GK treatment. The center has gathered 250,000 MR images of before and after GKS of 6 times follow-ups. Most of the VS will shrink after two years of GK, but 52% of patients will encounter pseudo-progression, that VS is temporally swelling and afterword reducing, after GK surgery. Recently, the factors for predicting prognosis after the GK is still unclear. Furthermore, the high heterogeneity, and different tissue composition, growth rate, patterns in MR images, and response to GK of VS are observed in clinical practic. Therefore, exploring the effective predictors for pseudo-progression and efficient shrinkage after GK surgery is a key issue of clinical research in VS. This project is proposed to conduct in two years, and consist of three subprojects. The sub-project 1 is responsible for collecting and labelling clinical data of VS for sub-project 2 and 3, discussing prognostic factors after GK, and verifying the appropriateness of the prediction model developed from sub-project 2 and 3; sub-project 2 is responsible for automatically detecting VS’s lesion and surrounding tissue and building a prognostic prediction model for GK; sub-project 3 will analyze the radiomics of the image of VS, select the clinically significant radiomics features, and integrate the radiomics features into the prognosis prediction model for GK. The PI of main project and sub-project 2 (AI team) and the PI of sub-project 3 (radiomics team) are experienced scientists in MR principles, image processing and analysis of various brain diseases. They have cooperated closely with the team of sub-project 1 (clinical data team in TVGH) for more than 10 years and understand the clinical language that enables them to quickly catch the clinical insights and tackle the problem by appropriate approaches. The salient features of this project is the integration of selected dominate feature maps and image biomarkers resulted from convolutional neural network and radiomics, respectively, on MR images of multiple follow-ups. The aim is to construct two prediction models to assist clinical decisions: one is to predict the possible outcome before GK and suggest whether the GK should be conducted, another is to predict the change of VS volume (3 categories: volume changes 10% and volume reduces > 10% ) and side effect of hearing damage (3 to 5 categories from mild to severe) after the second year after GK. Subsequently, the models will assist the treatment planning of GK surgery and improve the development of precision medicine.
Vestibular schwannoma (VS) is a benign tumor with slow growth rate and clear boundary. Gamma knife (GK) surgery is a promising treatment for patients with VS. Taipei Veterans General Hospital (TVGH) is an internationally renowned treatment center for its reputation of GK. Thousands of GK cases have been collected in TVGH, including 500 VS cases of complete GK treatment. The center has gathered 250,000 MR images of before and after GKS of 6 times follow-ups. Most of the VS will shrink after two years of GK, but 52% of patients will encounter pseudo-progression, that VS is temporally swelling and afterword reducing, after GK surgery. Recently, the factors for predicting prognosis after the GK is still unclear. Furthermore, the high heterogeneity, and different tissue composition, growth rate, patterns in MR images, and response to GK of VS are observed in clinical practic. Therefore, exploring the effective predictors for pseudo-progression and efficient shrinkage after GK surgery is a key issue of clinical research in VS. This project is proposed to conduct in two years, and consist of three subprojects. The sub-project 1 is responsible for collecting and labelling clinical data of VS for sub-project 2 and 3, discussing prognostic factors after GK, and verifying the appropriateness of the prediction model developed from sub-project 2 and 3; sub-project 2 is responsible for automatically detecting VS’s lesion and surrounding tissue and building a prognostic prediction model for GK; sub-project 3 will analyze the radiomics of the image of VS, select the clinically significant radiomics features, and integrate the radiomics features into the prognosis prediction model for GK. The PI of main project and sub-project 2 (AI team) and the PI of sub-project 3 (radiomics team) are experienced scientists in MR principles, image processing and analysis of various brain diseases. They have cooperated closely with the team of sub-project 1 (clinical data team in TVGH) for more than 10 years and understand the clinical language that enables them to quickly catch the clinical insights and tackle the problem by appropriate approaches. The salient features of this project is the integration of selected dominate feature maps and image biomarkers resulted from convolutional neural network and radiomics, respectively, on MR images of multiple follow-ups. The aim is to construct two prediction models to assist clinical decisions: one is to predict the possible outcome before GK and suggest whether the GK should be conducted, another is to predict the change of VS volume (3 categories: volume changes 10% and volume reduces > 10% ) and side effect of hearing damage (3 to 5 categories from mild to severe) after the second year after GK. Subsequently, the models will assist the treatment planning of GK surgery and improve the development of precision medicine.
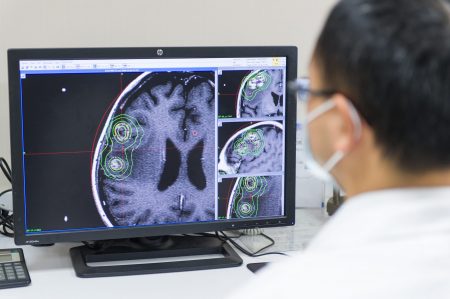
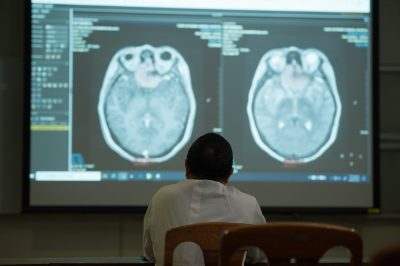
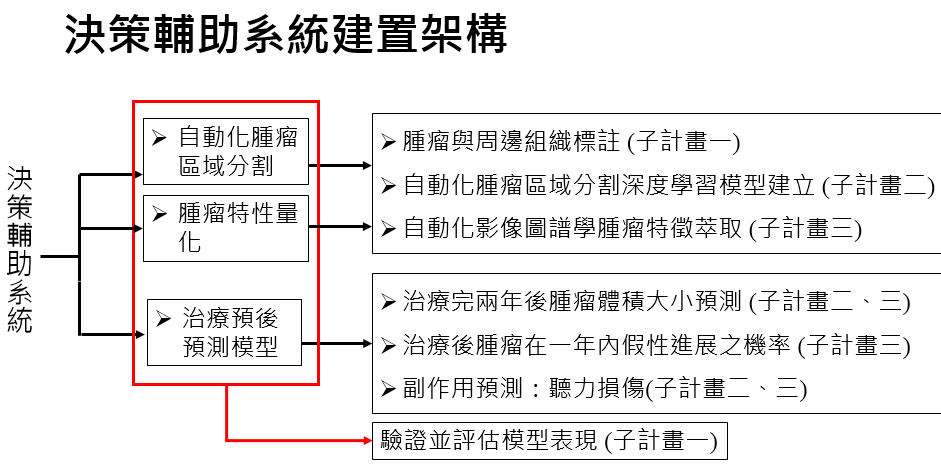
System Capabilities
- Multi-parameter MRI alignment & preprocessing platform
- Automatic pre & post surgery segmentation of acoustic neuroma tumors and cysts which may be used as reference for prognosis.
- Prediction of radiosurgery efficacy and side-effect evaluation using radiomics. It can be used to classify whether patient is responding to treatment, and to classify the two sub-types of response to treatment.
Cooperating Partner
- Taipei Veterans General Hospital
Contact info
Tel :02-2826-7169
Mail:mailto:ytwu@ym.edu.tw
Tel :02-2826-7169
Mail:mailto:ytwu@ym.edu.tw